How DeepSeek Rewrote the Rules of AI
An overlooked tool has helped launch an international race.
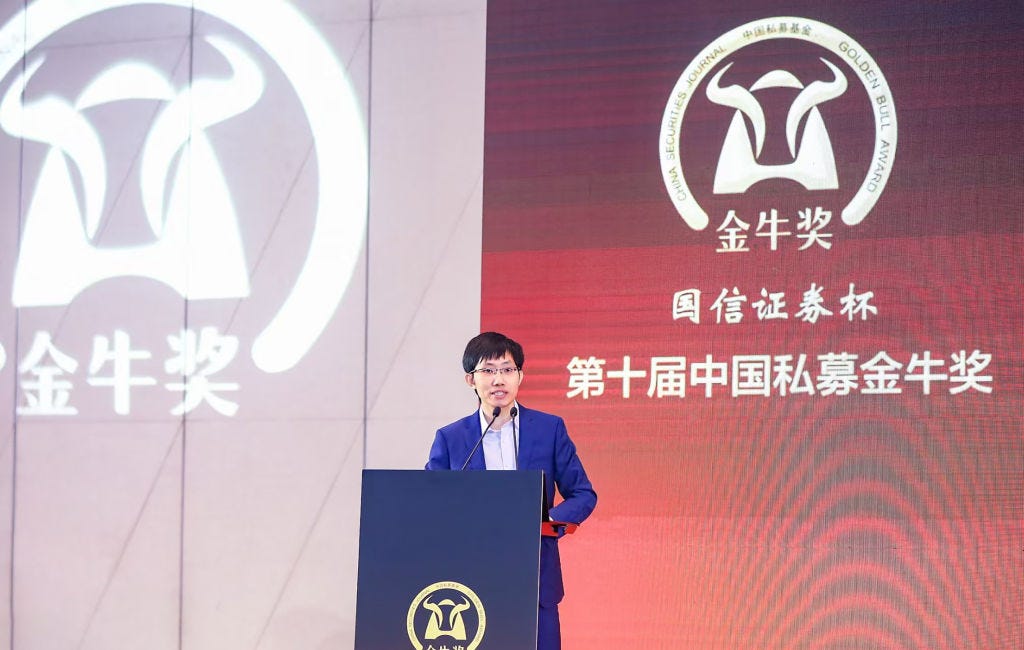
For years, distillation has been a workhorse behind the scenes in the AI community—a method by which a large, resource-intensive model’s knowledge is compressed into a leaner, faster system.
The technique, first demonstrated by Bucilua and collaborators in 2006 and later formalized by Hinton in 2014, follows a simple principle: a smaller “student” model learns to mimic a larger “teacher,” preserving the essential capabilities while dramatically reducing computational demands. It’s been a standard tool in the AI developer’s toolkit, quietly enabling everything from mobile speech recognition to efficient image processing. But last month, that routine optimization technique became the center of a storm that wiped $593 billion off Nvidia’s market value in a single day—the largest one-day loss for any company in Wall Street history, sending shockwaves through the tech sector and beyond.
The catalyst? A Chinese startup called DeepSeek that allegedly turned distillation from an optimization technique into a competitive weapon. The White House’s AI Czar David Sacks claimed there was “substantial evidence” that DeepSeek had systematically bombarded OpenAI’s chatbots with queries, harvesting responses to train its own model—a practice that goes far beyond traditional distillation’s accepted boundaries. OpenAI followed with accusations that DeepSeek “may have inappropriately distilled our models,” promising "aggressive, proactive countermeasures" to protect their technology.
But the legal battle that’s brewing may already be beside the point. OpenAI has limited recourse under either intellectual property or contract law. “There's a huge question in intellectual property law right now about whether the outputs of a generative AI can ever constitute creative expression or if they are necessarily unprotected facts,” explains Mason Kortz of Harvard Law School. Even if OpenAI could prove copying, their path forward is murky. The company’s own arguments in its ongoing battle with The New York Times—that training AI models falls under “fair use”—could undermine any case against DeepSeek. More fundamentally, the enforceability of AI terms of service across international borders remains largely untested.
While lawyers debate these points, the market has already rendered its verdict. DeepSeek claims it trained its competitive model for just $5.6 million in computing costs—a figure that Bernstein analyst Stacy Rasgon called “categorically false,” noting that the infrastructure and processing power required for advanced AI development typically runs into hundreds of millions. But whether the true number is $5 million or $50 million or more (and whether DeepSeek may have benefited more from the largesse of the Chinese state than has been acknowledged), it’s clear that DeepSeek has demonstrated something profound: the capital requirements for competitive AI development might be far lower than previously thought, especially when efficiency is prioritized over raw computing power.
This efficiency revolution wasn’t just clever engineering—it was born of necessity. When the Biden administration imposed chip embargoes in 2022, cutting off Chinese firms from advanced Nvidia AI semiconductors, companies like DeepSeek were forced to maximize the efficiency of older, lower-tier processors. What started as a workaround to hardware constraints evolved into a fundamental rethinking of model training. As Kai-Fu Lee, former president of Google China, observed, “When you have limited compute power and money, you learn how to build things very efficiently.” DeepSeek’s approach suggests that the future of AI might not lie in ever-larger models requiring massive computing resources, but in finding ingenious ways to do more with less.
The implications are already rippling through the industry. OpenAI has accelerated the release of its lightweight O3 model and Deep Research, offering premium capabilities at a fraction of the previous cost. This isn’t just another product launch—it’s a defensive move that signals a fundamental shift in the market. The question now isn’t just about technical capability but about value proposition: Will consumers continue paying $200 monthly for capabilities that are becoming more widely available? The economics that underpinned the AI boom—massive capital investment yielding exclusive capabilities—may be unraveling faster than anyone anticipated. And with that goes the assumption—which should have been more carefully examined than it had been—that the U.S. would be the one to call the shots in the AI boom, much in the way that Silicon Valley for decades reigned over the tech revolution and provided its immense boost to the U.S. economy.
Ironically, the trade restrictions meant to slow China’s AI progress may have forced China to innovate and to more decisively break the U.S.’ hold over AI. As Paul Triolo of DGA-Albright Stonebridge Group notes, “DeepSeek has thrown down quite a challenge to the AI community and the U.S. government.” The widespread adoption of DeepSeek’s open-source model could reshape the industry in ways that export controls never anticipated. More than just bypassing restrictions, DeepSeek’s approach suggests a new paradigm where innovation emerges not from the application of brute-force resources but from creative constraints.
The limitations of export controls become clearer in this context. Rather than preventing Chinese AI development, these restrictions may have inadvertently accelerated a shift toward efficiency that could prove more disruptive than raw computing power. This highlights a recurring challenge in trade policy: restrictions often drive innovation in unexpected directions, potentially leaving the restricting country fighting yesterday’s war. The real risk isn’t just losing a trade war—it’s missing the emergence of entirely new approaches to technological problems.
As the AI arms race transforms, American companies like OpenAI and Anthropic now find themselves committed to pushing the boundaries of raw capability, while DeepSeek’s efficiency-focused approach suggests the next breakthroughs may come not from computational power but from innovative methods of optimization and deployment. For consumers and smaller companies, this could mean access to AI capabilities that were once the exclusive domain of tech giants—a shift towards bottom-up technologies reminiscent of the rise of personal computing in the late ’70s at the expense of existing tech behemoths.
In the end, the DeepSeek controversy may be remembered less for its legal implications than for how it reshaped the economics of AI development. Whether through legitimate innovation or controversial methods, they’ve demonstrated that the barrier to entry in advanced AI might be lower than anyone imagined. As the industry grapples with this new reality, the winners are likely not those who build the most powerful models but those who deploy them most efficiently—and the biggest winners of all might be the consumers who benefit from this unexpected revolution in AI economics, one that transforms today’s premium capabilities into tomorrow’s commodities.
Nick Potkalitsky writes about artificial intelligence and education on his Substack Educating AI. An AI researcher and educator with a Ph.D. in new media, narrative, and rhetoric, he is the co-author, with Mike Kentz, of AI in Education: A Roadmap to a Teacher-Led Transformation.
Follow Persuasion on X, LinkedIn, and YouTube to keep up with our latest articles, podcasts, and events, as well as updates from excellent writers across our network.
And, to receive pieces like this in your inbox and support our work, subscribe below: